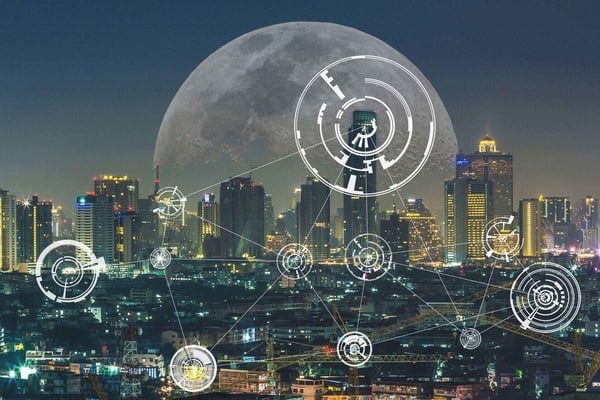
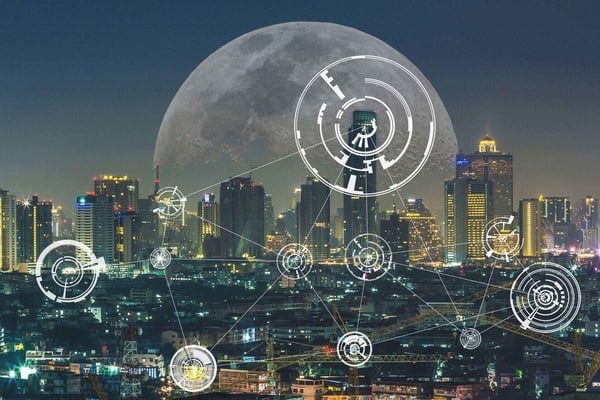
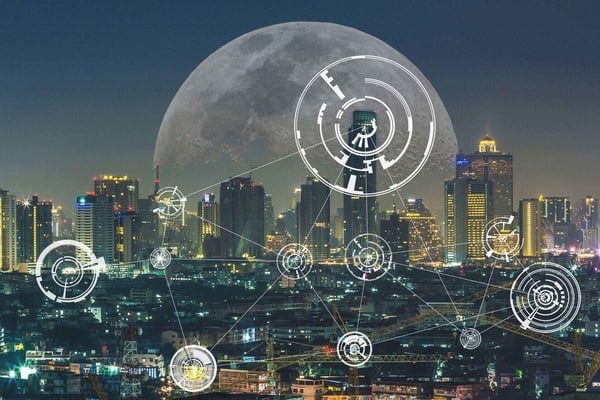
Ambient Computing – The Smart World
July 25, 2019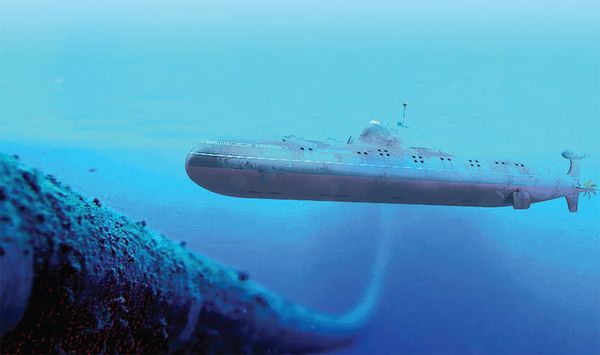
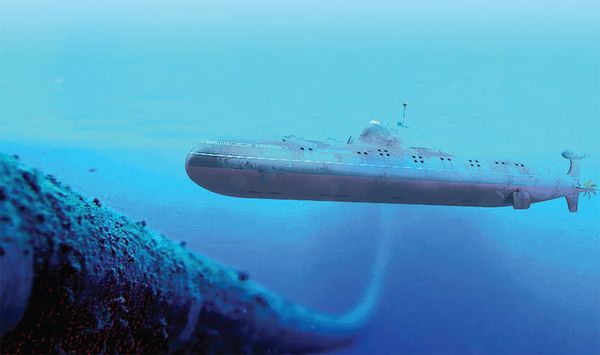
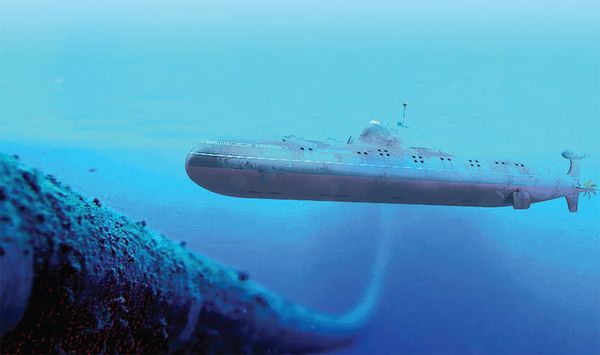
How Data Center Design Is Becoming More Resilient for Smoother Data Transfer
August 1, 2019Deep Learning and Machine Learning are often considered synonymous. Deep Learning is, in fact, a specialized form of Machine Learning that teaches computers to do what comes to humans naturally, i.e., learning by example. In Deep Learning, a computer model learns to perform classification tasks using data in the form of texts, images, or sound. The computer model is trained using large sets of labeled data and neural network architectures containing many layers.
With Deep Learning, we can achieve an accuracy that often exceeds human-level performance. Deep learning may have been conceptualized back in the 1980s, it was only recently that we began applying it to different industry verticals. This is mainly because the technology requires large sets of data and massive computing power in the form of GPUs or Graphics Processing Units.
Deep learning performs end-to-end learning where a computer is provided vast sets of raw data and a classification task to perform, and the job is completed automatically without any supervision. While machine learning requires you to choose features and a classifier to sort images, deep learning carries out feature extraction and modeling on its own.
Nowadays, Deep Learning finds applications in spheres ranging from driverless cars, image recognition, restoring colors in black and white images, real-time behavioral analysis and translation to reading the text in videos, robotics, voice generation, and even music composition. So why should data centers be an exception?
Read: 30 Amazing Applications of Deep Learning
The rising popularity of cloud-based services has resulted in the mushrooming of large-scale data centers. These data centers face an array of operational challenges on a day-to-day basis. One such problem is power management. Growing environmental awareness has put tremendous pressure on the data center industry to better its operational efficiency. As per current stats, data centers are using 2% of the energy produced globally. Even a slight reduction in this energy consumption can result in significant savings.
A large-scale data center generates millions of data points across numerous sensors every single day. This data is hardly used for any purpose other than monitoring. Now that we have advanced processing power and monitoring capabilities, we can leverage deep learning capabilities to improve data center efficiency and guide best practices.
Because of the complexity of operations and the massive amount of monitoring data available in a data center, in-depth learning solutions are ideal for optimizing data center operations. Every data center has a wide variety of mechanical and electrical equipment along with their control schemes and set points. The interaction between these systems makes it challenging to monitor the data centers using traditional engineering techniques. Use of predictive modeling techniques often results in significant errors on account of the complexities involved.
In a typical large-scale data center, there are many possible combinations of hardware and software that make it difficult to ascertain how efficiency can be attained. Testing each combination would be difficult if not impossible, given time constraints, fluctuations in workload, and the need to maintain a stable server environment.
To address the issues of complexity and abundance of data, in-depth learning solutions are applied wherein neural networks are chosen as the framework for training data center efficiency models. Neural networks are algorithms modeled loosely on the human brain that are designed to recognize patterns. They are suitable for modeling complex systems as they prevent the need to define feature interactions that assume relationships within the data. The neural network automatically searches the patterns and interactions between features to create a best-fit model.
Read: Transforming Cooling Optimization for Green Data Center via Deep Reinforcement Learning
Today, deep learning models are being tested to enhance every facet of data center management, including planning and design, managing fluctuating workloads, ensuring uptime, and reducing energy emission.
Examples of Smart Data Centers
Capacity Planning
With their capability to forecast demand accurately, neural networks can be used for capacity planning to ensure data centers do not run out of electricity, power, cooling, or space. Capacity planning can be of immense help to organizations that are setting up a new data center or relocating an existing one.
Considering the energy loss data centers face, it is imperative that accurate prediction models be developed using deep learning techniques. Precise capacity planning can help data centers decide how much of each resource is needed for optimal energy usage: how many servers are required or how much cooling power and electricity are needed to run theses servers at optimal capacity.
Deep learning algorithms can also be used to decide the most efficient way of configuring a data center by determining the physical placement of servers and other hardware equipment.
Optimization of Energy Consumption
As discussed above, all large-scale data centers act as energy hogs. Data centers are facing pressure from environmentalists to reduce their carbon emissions. In-depth learning solutions can be used to optimize cooling inside data center facilities by monitoring variables such as air temperature, power load, and air pressure in the back of servers.
Deep learning algorithms can study IT infrastructure to detect which servers are running at how much workload so that high workload can be shifted to newer, energy-efficient servers leading to an overall reduction in energy consumption. These algorithms can also be used to determine the best possible time to perform a task.
Prevention of Downtime
With their unique ability to detect patterns in massive sets of data, neural networks can be used to detect anomalies in the performance data that would be difficult to detect otherwise.
Deep learning models can be used to analyze data from equipment such as cooling systems to predict when they might fail. This information can be used to schedule preventive maintenance that can improve the shelf life of the equipment. Besides, the clients can be notified beforehand so that they can create a backup before downtime occurs.
Deep learning can also be used to model different configurations of the data center to improve its resilience. If downtime occurs, algorithms can be used to detect the root cause of failure faster.
Data centers are burgeoning, and so are similar operational issues. It is high time we switched from traditional data analyses to emerging technologies like machine learning and deep learning for enhancing operational efficiency and mitigating risks.