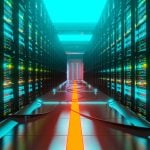
Data Center Usage by Major Companies: Why They Need New Facilities When There Are Empty Ones
July 13, 2023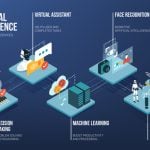
What Infrastructure Challenges Do AI and Big Data Face?
April 10, 2024On the Page
- What Are AI Hallucinations?
- Is Artificial Intelligence Really Hallucinating?
- What Is Batch Learning and Online Learning?
- What Is Instance-Based Learning and Model-Based Learning?
- Why Are AI Systems Hallucinating?
- How to Build Trustworthy AI Systems in the Future?
- Conclusion
What Are AI Hallucinations?
Artificial intelligence has been making strides at an unprecedented rate. While the technological advancements of AI have been nothing short of impressive, it has brought along a rather strange phenomenon known as “AI hallucinations”. These are moments where AI systems imagine things that are not real. Just like how we sometimes daydream, these systems can also have their own little ‘daydreams’, which are sometimes funny and at other times, quite odd. Let’s take a deeper look at what AI hallucinations are, their implications, and potential strategies to address this growing concern in the AI sector.
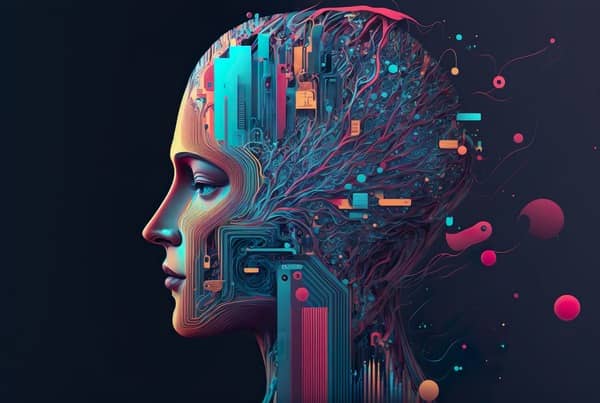
Is Artificial Intelligence Really Hallucinating?
AI hallucinations might sound like something you’d hear in a sci-fi movie, but it’s a real issue in the world of artificial intelligence right now. These AI hallucinations occur when artificial intelligence systems guess or create images that aren’t grounded in real facts. It seems as though the machines are letting their ‘imagination’ run wild, crafting images or descriptions that can be either amusing or outright incorrect.
This unpredictability can be tricky to navigate. On one hand, it can lead to amusing and even creatively inspiring results, showcasing the AI’s ability to ‘think’ outside the box. On the other hand, it can present a considerable challenge in ensuring the reliability and accuracy of AI systems. These hallucinations can potentially cause misinformation to spread, leading to confusion or mistrust among users. In more serious scenarios, if used in critical systems, it might result in incorrect decisions or analyses, which can potentially create additional problems in an organization.
It’s essential to handle these AI hallucinations carefully. As we continue to depend on AI for various tasks, it becomes increasingly important to develop mechanisms that can prevent these hallucinations or at least minimize their negative impact. It emphasizes the need for us to build better and more reliable tools to ensure AI remains safe and trustworthy, avoiding the pitfalls of machines letting their ‘imaginations’ run too wild.
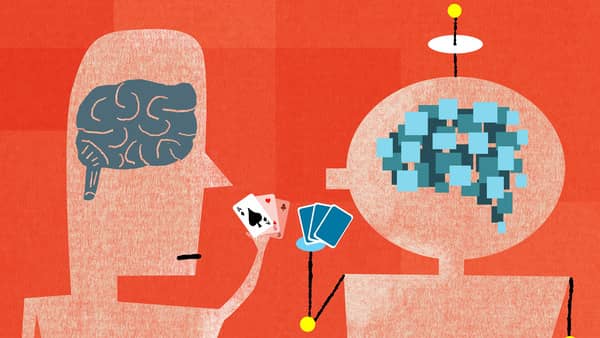
What Is Batch Learning and Online Learning?
To fully grasp why AI hallucinations, happen, we need to understand how AI learns. There are different learning methods, including batch learning and online learning, which come with their own sets of strengths and weaknesses.
In Batch Learning, all the training data is processed in one big chunk, offline. It’s usually used when the data doesn’t change much over time and needs a lot of computing power. It’s great for things like understanding languages or recognizing images, but it struggles to adapt to new, changing data without being retrained from scratch. Batch learning is also known for its stability and reliability in predictions, as it is less likely to be swayed by outliers or noise in the data. However, it can be time-consuming and require significant computational resources, especially with larger datasets.
On the other hand, Online Learning happens in real-time, where the model learns and adapts as it receives new data. This means it can potentially be good at dealing with data that keeps changing, quickly adapting to any new patterns it notices. It’s often used in fields like financial market analysis, where it helps predict market trends, or in recommendation systems that suggest products to users based on their browsing history.
Online learning can offer more personalized results and can be more scalable to large datasets. However, it may sometimes prioritize speed over accuracy, potentially leading to less reliable predictions if not monitored and adjusted appropriately. It can also be more vulnerable to data anomalies, incorporating them into the model which might affect its performance negatively.
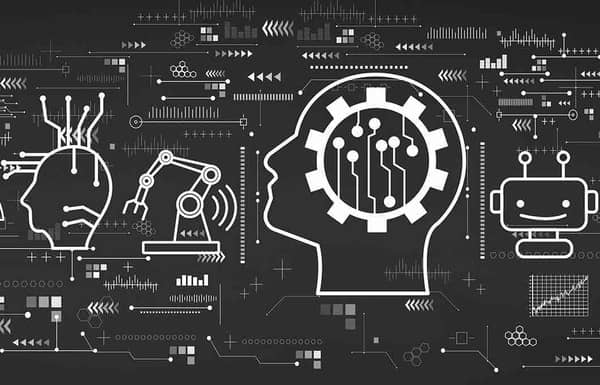
What Is Instance-Based Learning and Model-Based Learning?
As we go further into how AI systems learn, we find other learning methods like instance-based and model-based learning, which play a huge part in how AI learns and sometimes, ‘hallucinates’.
Instance-based learning functions somewhat like a detailed scrapbook. This learning method memorizes specific examples from the training data and uses these to make informed predictions when faced with new, unseen data, essentially comparing new inputs with stored memories to find similarities. This approach can quickly adapt to new data and works well with smaller, complicated datasets. However, it requires a substantial amount of memory and can have difficulty processing larger datasets, needing to initiate a new analysis for each query, which can be time-consuming.
On the other side, model-based learning operates like constructing a comprehensive guidebook. It looks to develop a general understanding or “model” of the data’s patterns and relationships, serving as a blueprint when making predictions or decisions. This method can effectively generalize new scenarios, encapsulate complex relationships, and handle large datasets adeptly. However, it requires a considerable amount of computational power and time for training, potentially faltering when the data isn’t organized properly.
Both methods aim to make precise predictions using training examples, offering diverse pathways to achieve this goal in the ever-evolving landscape of AI learning.
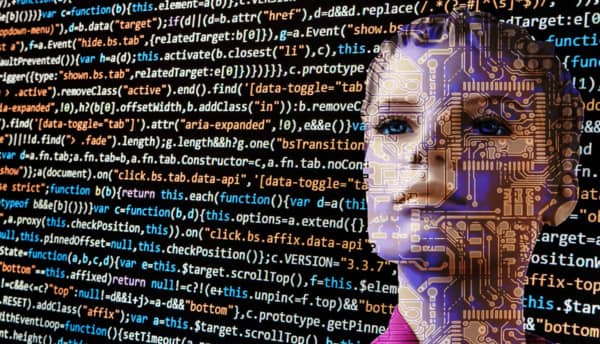
Why Are AI Systems Hallucinating?
AI hallucinations, where systems that use large language models fabricate or generate incorrect information, deviating from the actual or expected outcomes, are a cause for concern. Experts warn that this issue, stemming from the fundamental operation of models like ChatGPT, might not be easily resolved. These models essentially predict the next word in a sequence based on a given prompt, without truly comprehending the context or meaning of the words, therefore accuracy in their responses often comes by chance, leading to the generation of incorrect or misleading information.
The black-box nature of many AI systems worsens this problem, as users are unable to understand the reasoning or data used to reach a particular conclusion, developing an environment where AI systems are not held accountable for inaccurate outcomes. However, there is hope for a more trustworthy AI future with the emergence of frameworks like Instance-Based Learning. IBL allows for a more transparent and accountable AI decision-making process, by tracing every decision back to the specific training data that influenced it, offering a potential pathway to reduce hallucinations in AI systems.
By handling these fundamental challenges, we can develop a future where AI systems are more reliable, trustworthy, and aligned with values of accuracy and integrity. This endeavor mandates a concerted effort to lessen the risks associated with the current generation of AI models, promoting a safer and more responsible AI landscape.
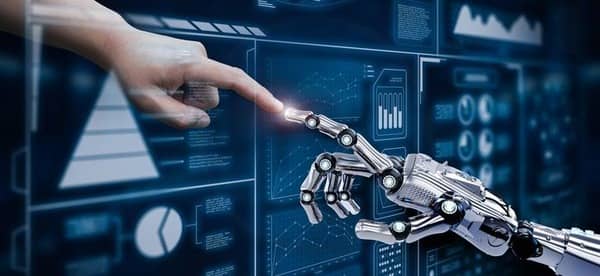
How to Build Trustworthy AI Systems in the Future?
To make AI systems we can trust, combining the best parts of the learning methods we discussed above will be important. This balanced approach could help create AI systems that are more reliable, avoiding hallucinations by checking and cross-checking data from different angles, and making sure the predictions and decisions are based on real facts.
It’s important to build AI models that can show us how they arrived at a certain decision, using clear paths that can be traced back to the original data. This way, the AI remains responsible, and we can trust it to make important decisions without fearing hallucinations.
Conclusion
As artificial intelligence solidifies its presence in our daily lives, it’s crucial to acknowledge and address the unique phenomenon of AI hallucinations, which can be both amusing and a cause for caution. It is essential that as we further develop AI technology, we do so with a commitment to accuracy and reliability, to prevent the inception of these hallucinations. By integrating the strengths of various learning models and following stringent guidelines, we can pave the way for AI systems that are not only grounded in facts but also dependable in our technological progression.