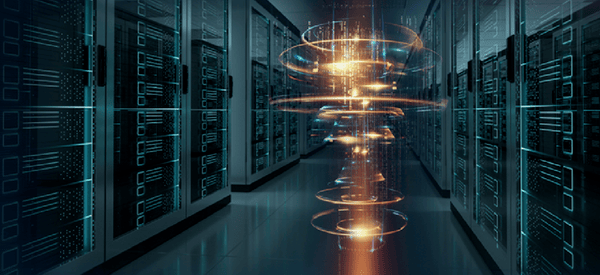
How Quantum Computing Will Help Data Center Operations
January 23, 2023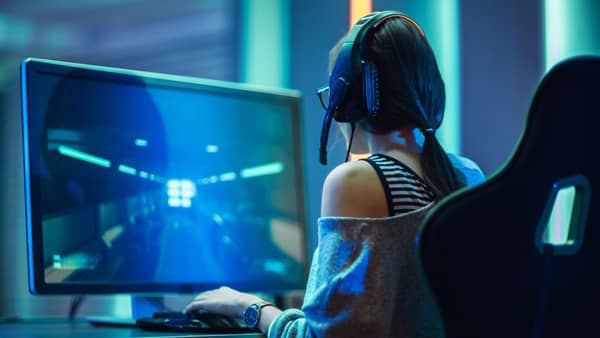
How Does Virtualization Affect Gaming Performance?
February 3, 2023Artificial intelligent technology has come a long way in recent years. Deep learning and machine learning are both advancements in artificial intelligence used to analyze and interpret data. Machine learning is a broader field that encompasses a variety of techniques. In contrast, deep learning is a specific type of machine learning that uses artificial neural networks with multiple layers to learn and make decisions. Under the umbrella of deep learning is an innovation called the text-to-image model. We will explore the deep learning text-to-image model, its wide range of potential applications, and what it could potentially mean for all users on the internet.
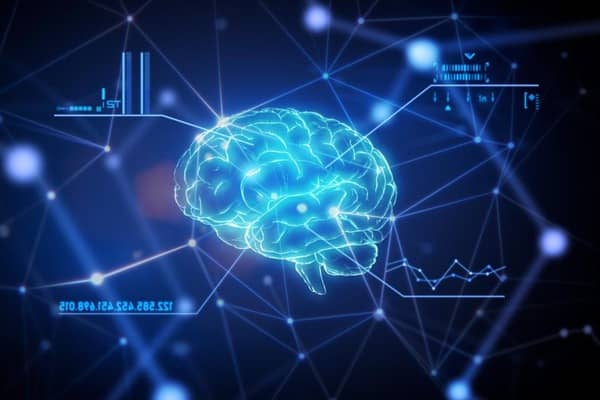
What Is the Text-to-Image Model?
The deep learning text-to-image model is a machine learning algorithm that uses neural networks to generate images from text descriptions. These models can learn the association between the text and the image data and generate new images based on the inputted prompt.
The architecture of a deep learning text-to-image model regularly involves two main parts, which include a text encoder and an image generator. The text encoder converts the inputted text prompt into a compact and meaningful representation, which is then delivered to the image generator to create a final image.
The text encoder can be implemented using several natural language processing techniques including a Recurrent Neural Network or a Transformer Network. The image generator can be employed by using different kinds of generative models such as Generative Adversarial Networks or Variational Autoencoders.
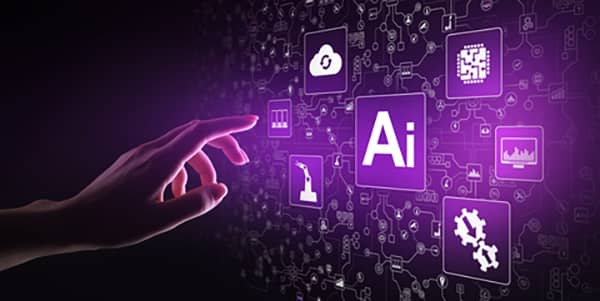
What Are the Applications of Deep Learning Text-to-Image?
Deep learning text-to-image models have a wide range of potential applications. One of the main applications is image generation, where the model generates images based on the inputted text prompt. Because the model is trained on a dataset of images and their corresponding captions—it can generate new images of objects or scenes centered on the given prompt. This can be used to create realistic images of things that may not exist or to generate images of objects with specific attributes.
One of the most popular text-to-image AI generators is Stable Diffusion, also known as Dream Studio. Some text-to-image AI generators include AI Image Generator by Fotor, NightCafe, Dream by WOMBO, DALL-E 2, MidJourney, and Craiyon. AI image generators have been gaining more popularity in the past year.
Another application of deep learning text-to-image models is image captioning, where the model generates captions for images. Text-to-image models can be beneficial for accessibility, where images can be captioned to make them more understandable for the visually impaired.
Deep learning text-to-image models can also be used for visual question answering, where the model generates an image that answers a given visual question. The model can be asked to generate something like an image of a lion with three legs, and even though it may not exist in the real world, the model will generate an image of a lion with three legs as the output.
Text-to-image models can also be used in various industries such as gaming, animation, architecture, and fashion. The technology can be beneficial for most industries where the use of images is prevalent. The deep learning text-to-image models have a wide range of potential applications and can be used in various fields to generate images based on the prompt it’s given. It has the potential to save its users a lot of time, especially in the early stages of a given project.
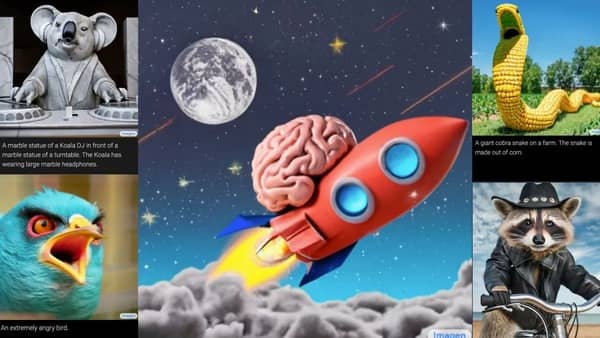
What Are the Challenges for Text-to-Image Technology?
Deep learning text-to-image models, like most machine learning models, face several challenges. One of the main challenges is the availability and quality of the data it uses. Training these models requires a large dataset of images and their corresponding text descriptions, which can be challenging and potentially low quality. The model may be limited if the dataset isn’t diverse.
Another challenge is the difficulty in evaluating the quality of generated images. Since the model generates new images based on textual input, it may be difficult for the model to determine if the generated images are genuine or believable.
Text-to-image models also may not be able to generate images with high realism and diversity. The images generated by these models may lack important details. It may also be limited in variation.
Text-to-image models are still in the early stages of development and require more innovation. The models still face challenges like handling multiple styles of texts and images, especially concerning details.
While deep learning text-to-image models are showing great potential, there is still more advancements needed. Nonetheless, the technology may potentially make certain users question if the image they are looking at is true or false.
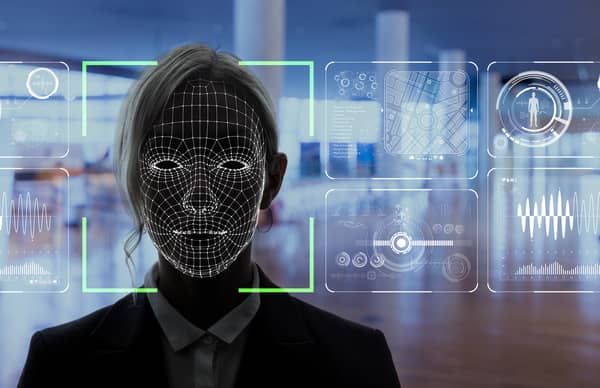
How Deep Learning Could Make the Internet Unreliable?
Deep learning can potentially be a powerful tool, but it also has the potential to make the internet unreliable in specific scenarios. One example is the possibility of deepfake technology to create false or misleading images and videos. These deep fakes can be difficult to detect and spread quickly on the internet, potentially causing confusion and mistrust.
It also has the potential for deep learning models to be used for clickbait or fake news generation. These models can be trained on existing clickbait and fake news and can generate new, similar content that can be spread on the internet, leading to the spread of misinformation. Deep learning can also be used for creating fake reviews, which can lead to unreliable information about products and services on e-commerce websites.
Although deep learning can improve the creation of images, it is crucial to be aware of the potential negative effects of text-to-image generators and to take steps to mitigate them. This includes developing techniques for detecting deepfakes and fake content, as well as promoting digital literacy to help users identify and evaluate sources of information.
Conclusion
Artificial intelligence, machine learning, and deep learning technologies are advancing in various ways. The deep learning text-to-image model is bringing in the next wave of content creation making it easier for organizations and individuals to create images for their benefit. This technology can also be beneficial for accessibility. While this technology has many upsides, there is also potential for the technology to be misused. As deep learning technologies advance, users should be aware of the potential on all sides. Nevertheless, artificial intelligence is advancing and looks to play a significant role in various industries.